Top 5 Challenges Data Analysts Face Today
Data analysts face numerous challenges in today’s data-driven world—large volumes, complexity, data silos, skill gaps, and data quality issues. Learn how technology and AI can help overcome these hurdles.
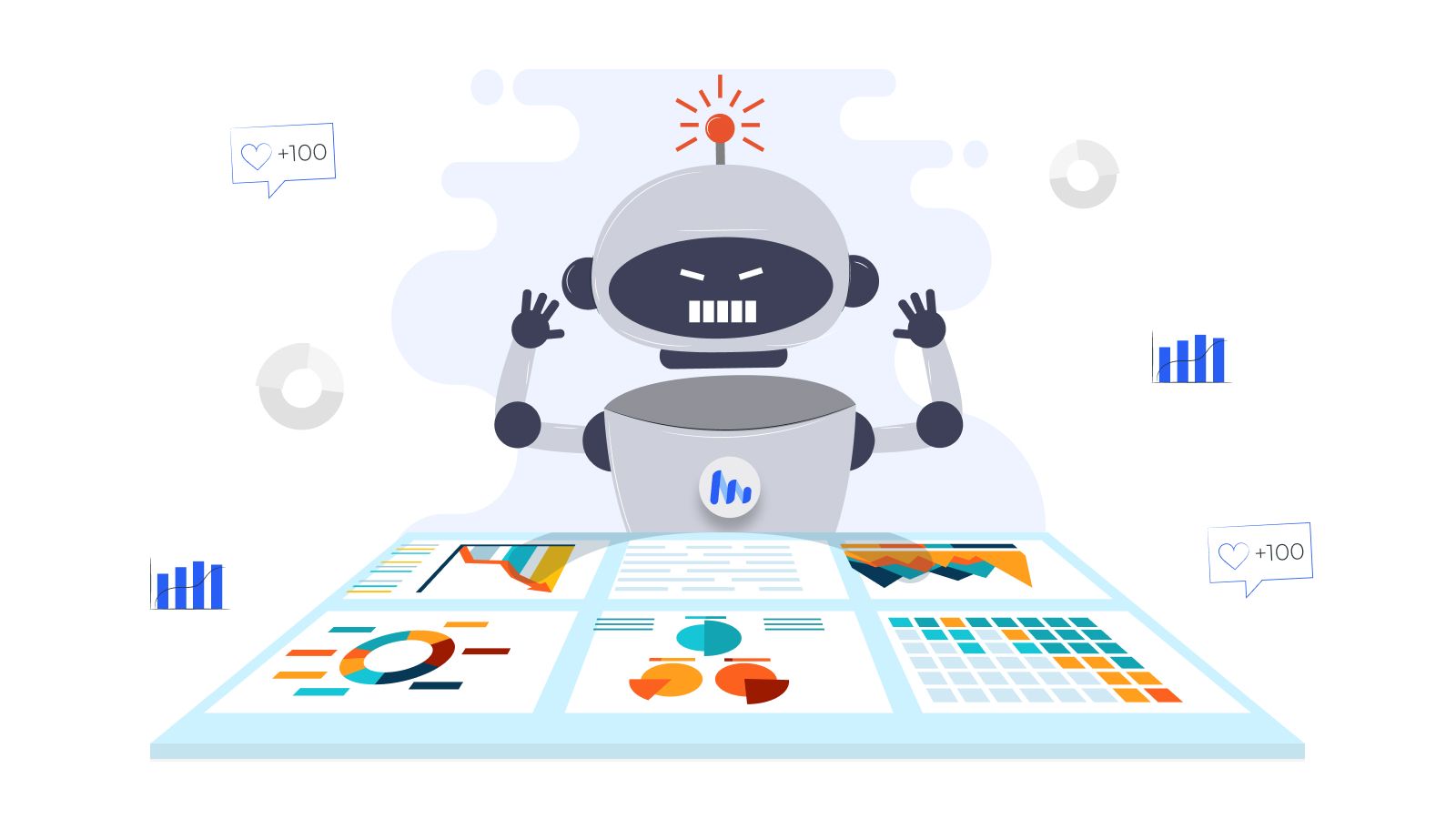
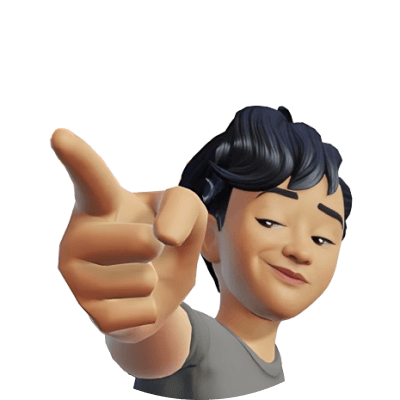
Rupesh Raj
2024-12-04
The role of data analysts is more critical than ever as companies increasingly rely on data to inform their decisions. But while data holds the key to valuable insights, it doesn’t come without its difficulties. Today’s data analysts navigate a landscape filled with rapidly expanding datasets, ever-more complex analytics, and the constant pressure to turn raw information into actionable intelligence. In this evolving world, there are a few significant hurdles data analysts face.
Let’s dive into the top five challenges that are reshaping the role of data analysts today.
1. Drowning in Data
Data is everywhere—and more of it is being generated every second. While the abundance of data should, in theory, provide richer insights, the sheer volume can actually feel overwhelming. Data analysts often find themselves spending more time wrangling data than analyzing it, which can be frustrating when deadlines loom and the demand for insights never slows down.
The tricky part isn’t just the amount of data but also figuring out which data is relevant. It’s like looking for a needle in a haystack, where the haystack keeps getting bigger by the minute. Having data pouring in from multiple sources—customer databases, social media, website analytics—means analysts need to decide what’s valuable and what’s just noise.
2. Increasing Complexity of Data Sources
It’s not just the volume that’s growing—data is becoming more diverse and complex. Gone are the days when analysts worked solely with neat rows of numbers in spreadsheets. Now, data can be anything from video files and customer reviews to social media posts and voice recordings. The challenge here? Making sense of all this unstructured data. Traditional tools weren’t built for this kind of diversity, and that’s where data analysts hit roadblocks. Analyzing this data requires new approaches, which means learning new skills, adopting advanced tools, and sometimes even collaborating with machine learning experts to build models that can decipher complex patterns.
3. The Silo Effect: When Data Doesn’t Communicate
Data silos remain one of the most persistent challenges for organizations. Departments collect and manage their own data, but they don’t always share it. For a data analyst, this means incomplete insights and potentially misleading conclusions. Imagine trying to build a customer behavior model without knowing about customer support interactions or financial transactions—it’s like solving a puzzle with half the pieces missing. Data analysts today need to work around these silos, either by manually piecing together fragmented data or pushing their organizations to embrace more integrated data management practices. Cross-department collaboration is key, but it’s not always easy to achieve.
4. The Need for a Broader Skillset
The role of a data analyst used to be fairly straightforward: crunch the numbers, build reports, and deliver findings. Today, that job description has ballooned. Analysts are expected to be proficient not only in Excel but also in advanced tools like Python, R, SQL, and even machine learning algorithms. The learning curve can be steep. The rise of self-service analytics tools and the increasing need for data visualization means that analysts also need to be excellent storytellers. It’s no longer enough to present numbers—you need to explain them in a way that makes sense to business leaders who may not have a background in analytics. Communicating insights visually and clearly is now an essential part of the job, adding yet another layer to the analyst’s toolkit.
5. Data Quality: Garbage In, Garbage Out
No matter how skilled an analyst is, poor data will lead to poor insights. Data quality remains a constant headache—whether it’s missing values, inconsistencies, or inaccuracies. Even small errors can skew results and lead to misguided business decisions. Fixing these issues, often referred to as data “cleaning,” can take up a significant portion of an analyst’s time.
It’s a tedious task, but essential to the process. Bad data in the system? That means unreliable conclusions. And when the stakes are high, from financial forecasts to product development decisions, data quality can make or break the outcomes.
How Analysts Can Navigate These Challenges
Let’s be honest: these challenges aren’t going away. However, there are ways data analysts can adapt and thrive in this ever-changing landscape.
Embrace Automation and AI Tools
With more data than any human could handle, automation is no longer optional—it’s necessary. AI-powered tools can sift through mountains of data, flagging patterns and anomalies without requiring an analyst to manually crunch every number. These tools can clean data faster, identify trends, and even generate predictive models, leaving analysts free to focus on interpreting and applying insights to real-world problems.
Prioritize Cross-Department Collaboration
Breaking down data silos is crucial. Analysts should actively work with other departments to ensure that data flows freely between teams. While this might require influencing higher management to invest in integrated data platforms, the payoff is worth it. A more complete dataset means more accurate insights and, ultimately, better decisions.
Continuous Learning is Key
The tools and techniques available to data analysts are constantly evolving. Staying ahead of the curve requires continuous learning. Whether through online courses, webinars, or simply staying active in data analytics communities, analysts need to keep updating their skillset. In particular, learning more about AI, machine learning, and advanced analytics will be critical for the future.
Improve Data Governance
Improving data quality starts with good data governance. By setting standards for how data is collected, managed, and maintained, organizations can drastically reduce the amount of cleaning that analysts need to do. Analysts can play a role here by advocating for better data management practices across their organizations.
Final Thoughts
The role of data analysts is rapidly evolving, and with that comes a new set of challenges. From the overwhelming volume of data to the increasing complexity of analytics, today’s data analysts are under more pressure than ever before. However, by embracing technology, fostering collaboration, and continuously honing their skills, they can turn these challenges into opportunities for growth and innovation. As data continues to grow in both volume and importance, overcoming these hurdles isn’t just about staying relevant—it’s about shaping the future of data-driven decision-making.
Related posts
Looking for a marketing purpose analytics tool?
Click HereNewsletter
Website owned by : KAIROS LABS PRIVATE LIMITED, Tonk Phatak Jaipur - 302015, Rajasthan
All Rights Reserved
Email : Support@llmate.ai