Retrieval Augmented Generation: Unlocking the Future of Content Creation
Discover how Retrieval Augmented Generation (RAG) is revolutionizing content creation by combining AI with real-world data retrieval. Explore its benefits, use cases, and future potential.
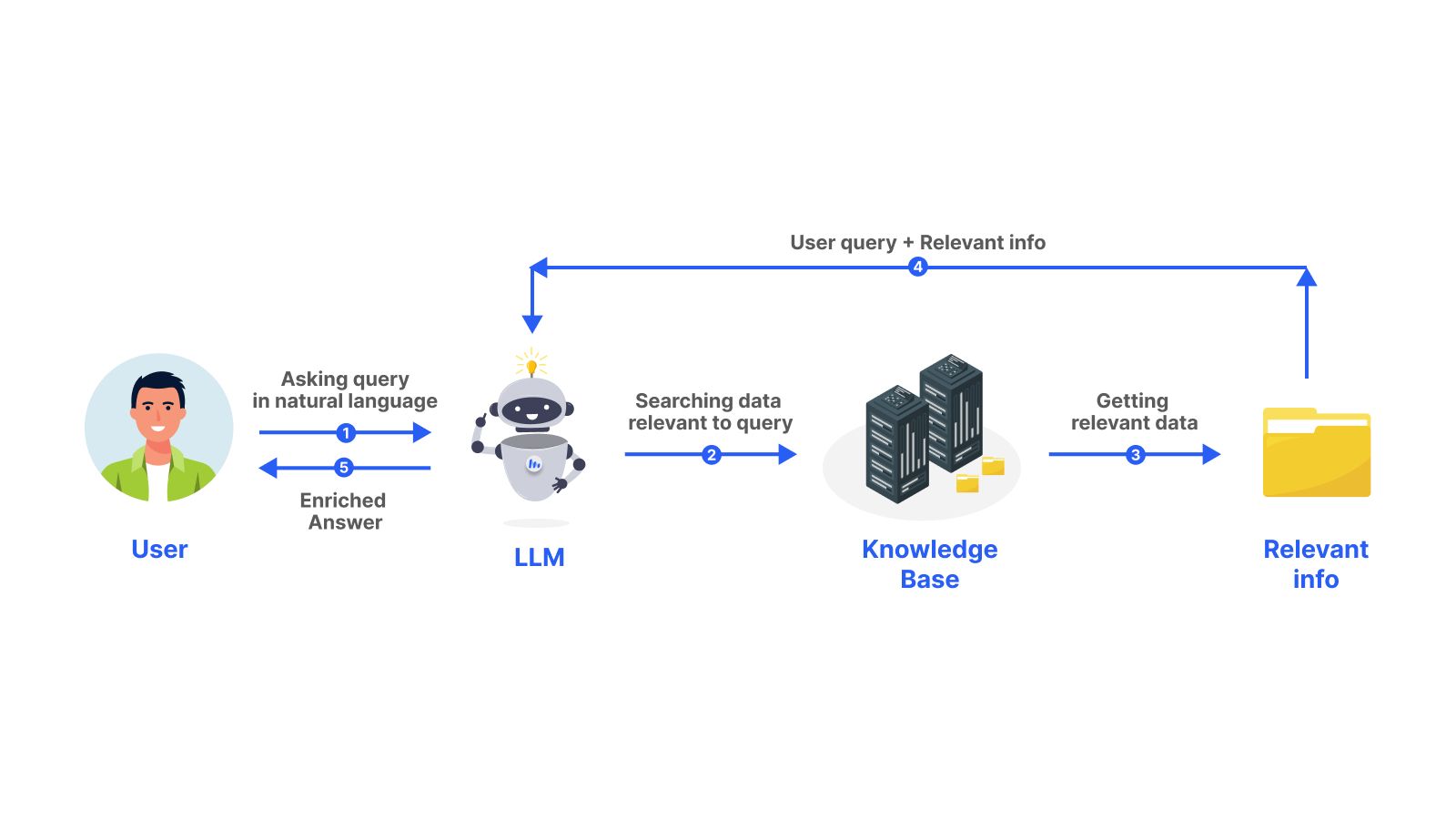
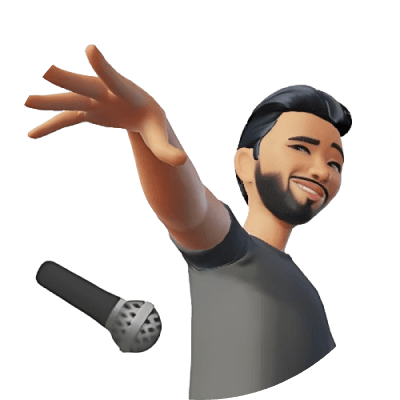
Nikhil Allamsetti
2024-12-05
In the fast-evolving digital landscape, the demand for high-quality, engaging and informative content is at an all-time high. As businesses and individuals seek to captivate their audience, one cutting-edge technology that's been making waves is Retrieval Augmented Generation. This innovative approach combines the power of AI-driven content generation with data retrieval to create content that is not only unique but also highly relevant to the user's needs.
What is Retrieval Augmented Generation?
Retrieval Augmented Generation (RAG) is a method of improving the accuracy and informativeness of language model outputs by providing them with access to relevant documents or data points. In simpler terms, it combines two main ways of responding to queries: retrieval-based modelling and generative modelling. It gathers relevant data and then generates a unique response based on that data.
Benefits of using RAG
Retrieval Augmented Generation (RAG) is a new and powerful technology with many benefits, including:
Improved Accuracy
RAG can help to improve the accuracy of content by providing LLMs with access to real-world information. This means that RAG-generated content is more likely to be factually correct and up-to-date. For example, if a blogger is writing an article about a new product, they can use RAG to access information about the product from the manufacturer's website, customer reviews and other reliable sources. This information can then be used to verify the accuracy of the article's content.
Reduced Hallucinations
Hallucinations are errors that can occur when LLMs are asked to generate content on topics that they are not familiar with. For example, if an LLM is asked to write a poem about a topic that it does not know anything about, it may generate a poem that is factually incorrect or nonsensical. RAG can help to reduce the risk of hallucinations by providing LLMs with access to real-world information. This means that RAG-generated content is more likely to be coherent and relevant to the topic at hand.
Increased Versatility
RAG can also help to increase the versatility of content creation. This is because it can be used to generate a wide variety of content formats, including blog posts, articles, product descriptions, marketing copy and more. For example, a business can use RAG to generate product descriptions for its website, blog posts to promote its products and social media posts to engage with its customers. RAG can also be used to generate personalized content for individual users.
Improved SEO
RAG-generated content is more likely to rank well in search engines because it is more relevant and informative. When RAG generates content, it takes into account the keywords and phrases that people are searching for online. This means that RAG-generated content is more likely to match the search intent of users, which can lead to higher rankings in search engine results pages (SERPs).
Use cases for RAG
Retrieval augmented generation (RAG) has a wide range of use cases, including:
1. Question Answering
RAG can be used to answer questions in a comprehensive and informative way. This is because it has access to a vast amount of information from the real world. For instance, if you ask RAG what the capital of France is, it will be able to look up the answer on reliable websites like Wikipedia and Britannica. It can then use this information to generate a comprehensive and informative answer, such as "The capital of France is Paris."
2. Summarization
RAG can also be used to summarize long texts into shorter, more concise versions. This is useful for tasks such as summarizing news articles, research papers and other types of documents. For example, if you ask RAG to summarize a long news article, it will be able to identify the key points of the article and generate a shorter version that captures the most important information.
3. Translation
RAG can also be used to translate text from one language to another. This is useful for tasks such as translating websites, documents and other types of content. For example, if you ask RAG to translate an English article into Spanish, it will be able to access information from sources such as Google Translate and other translation services. It can then use this information to generate a translation that is accurate and fluent.
4. Code Generation
RAG can also be used to generate code. This is useful for tasks such as developing software applications and creating websites. For example, if you ask RAG to generate a Python function to calculate the factorial of a number, it will be able to access information from sources such as GitHub and Stack Overflow. It can then use this information to generate a function that is correct and efficient.
5. Creative Writing
RAG can be used to generate creative content, such as poems, stories and scripts. It can access and process information from a wide range of sources, including books, movies and TV shows. It can then use this information to generate creative content that is both original and engaging.
6. Customer Service
RAG can be used to create customer service chatbots and virtual assistants. It can access and process information from a wide range of customer service resources, such as knowledge bases, FAQs and product documentation. It can then use this information to answer customer questions and resolve customer issues quickly and efficiently.
RAG and LLMATE Platform
LLMATE is redefining customer service with its cutting-edge AI chatbots driven by Retrieval Augmented Generation (RAG) technology. This innovative approach combines the best of retrieval-based and generative models, allowing chatbots to understand user queries more accurately and deliver comprehensive, informative responses.
Gone are the days of frustratingly limited chatbots that struggle to grasp complex questions or provide relevant information. LLMATE's RAG-powered bots leverage the vast knowledge stored within databases, enabling them to retrieve and synthesize accurate data tailored to each user's inquiry. This results in a more natural and human-like conversation, where customers receive the precise information they need without any unnecessary delays or frustrations.
Beyond simple data retrieval, LLMATE's chatbots utilize their generative capabilities to craft personalized responses that address the specific context of each query. This allows them to provide insightful recommendations, offer helpful advice and even engage in empathetic conversations that foster trust and build stronger customer relationships.
In conclusion, Retrieval Augmented Generation is a game-changer in the realm of natural language processing. It improves the accuracy of responses, reduces hallucinations and is versatile enough to be used in various industries. RAG is the future of content creation and it is here to stay. As the technology continues to evolve, we can expect to see more innovative applications of RAG in various industries.
Related posts
Looking for a marketing purpose analytics tool?
Click HereWebsite owned by : KAIROS LABS PRIVATE LIMITED, Tonk Phatak Jaipur - 302015, Rajasthan
All Rights Reserved
Email : Support@llmate.ai